Neuromorphic engineering advancements are setting the stage for a transformative leap in artificial intelligence (AI). This cutting-edge field draws inspiration from the human brain’s architecture and functionality, enabling the creation of highly efficient and adaptive AI systems. By mimicking biological neural networks, neuromorphic engineering is revolutionizing AI in areas such as pattern recognition, energy efficiency, and real-time learning.
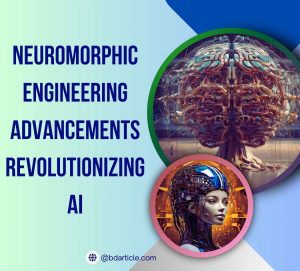
We’ll explore neuromorphic engineering’s breakthroughs, applications, and potential, shedding light on how it is reshaping the future of technology.
What is Neuromorphic Engineering?
Neuromorphic engineering is a multidisciplinary field that integrates neuroscience, computer science, and electrical engineering. The primary goal is to design hardware and software systems that replicate the brain’s neural networks. Unlike traditional computing systems, which rely on sequential processing, neuromorphic systems use parallel processing to achieve unparalleled efficiency and adaptability.
Neuromorphic chips, such as Intel’s Loihi and IBM’s TrueNorth, are examples of how this technology is applied. These chips simulate synaptic connections and spikes in neural activity, enabling faster and more energy-efficient data processing.
Key Neuromorphic Engineering Advancements
-
Neuromorphic Chips
The development of neuromorphic chips is a game-changer in AI. These chips are designed to process information in a way similar to the human brain, allowing for real-time processing and reduced energy consumption.
- Intel Loihi: A pioneering neuromorphic processor capable of learning and adapting in real-time without the need for extensive datasets.
- IBM TrueNorth: A chip with over one million neurons and 256 million synapses designed for pattern recognition and sensory data processing.
-
Event-Driven Processing
Neuromorphic systems utilize event-driven processing, where computations occur only when specific conditions are met. This significantly reduces energy consumption compared to conventional systems.
-
Dynamic Learning Capabilities
Neuromorphic engineering advancements enable systems to learn dynamically and adapt to new information, much like the human brain. This is especially useful in robotics, autonomous vehicles, and real-time analytics.
-
Low-Power AI Systems
One of the standout benefits of neuromorphic engineering is its ability to perform complex computations with minimal energy usage, making it ideal for IoT devices and wearable technology.
Applications of Neuromorphic Engineering in AI
-
Robotics and Automation
Neuromorphic systems enhance the capabilities of robots, enabling them to process sensory inputs and make decisions in real-time. Robots equipped with neuromorphic chips can navigate complex environments, recognize objects, and interact with humans more naturally.
-
Healthcare Technology
In the medical field, neuromorphic engineering advancements are driving innovations in diagnostic tools and prosthetics. AI-powered neuromorphic systems can analyze medical images, detect anomalies, and support real-time decision-making during surgeries.
-
Smart Cities
Neuromorphic systems are essential for the development of smart cities. From traffic management to energy optimization, these systems efficiently process data from numerous sensors, facilitating better urban planning and sustainability.
-
Edge Computing
Neuromorphic chips are highly suited for edge computing, where data is processed locally rather than relying on cloud computing. This is particularly beneficial for applications requiring low latency, such as autonomous vehicles and drones.
-
Security Systems
By integrating neuromorphic engineering, AI-driven security systems can recognize patterns in behavior and detect anomalies, improving threat detection and response times.
Challenges and Opportunities
Challenges
- Complexity of Development: Designing and manufacturing neuromorphic systems requires advanced expertise and significant resources.
- Scalability Issues: Scaling neuromorphic systems to meet the demands of large-scale applications remains a hurdle.
- Integration with Existing Systems: Adapting current AI systems to neuromorphic architectures poses compatibility challenges.
Opportunities
- Unmatched Efficiency: Neuromorphic systems offer energy-efficient solutions for AI applications, reducing operational costs.
- Real-Time Processing: Their ability to process data in real-time opens up new possibilities in robotics, healthcare, and beyond.
- Biological Computing: Advancements in neuromorphic engineering allow for the integration of biological components into computing systems, further blurring the line between technology and biology.
Exploring Micro-Business Funding Options
For startups and smaller enterprises venturing into neuromorphic engineering, securing funding can be a significant challenge. Exploring micro-business funding options, such as grants, angel investors, and government-backed initiatives, provides the financial support needed to drive innovation in this field. Platforms like Kickstarter and Indiegogo can also help entrepreneurs bring their ideas to life.
The Future of Neuromorphic Engineering in AI
Neuromorphic engineering advancements are poised to redefine the AI landscape. As the demand for intelligent, energy-efficient systems grows, neuromorphic technologies will play a pivotal role in meeting these needs.
-
Human-Machine Collaboration
The ability of neuromorphic systems to mimic human brain functions opens new avenues for collaboration between humans and machines, enhancing productivity across industries.
-
Personalized AI Systems
Neuromorphic chips can power personalized AI systems that learn and adapt to individual users’ preferences, improving user experiences in applications like virtual assistants and smart homes.
-
Breakthroughs in Neuroscience
By replicating the brain’s functions, neuromorphic engineering contributes valuable insights to neuroscience, aiding in the understanding of cognitive processes and neurological disorders.
Leveraging Neuromorphic Systems for Sustainability
Energy efficiency is a cornerstone of neuromorphic engineering advancements. By drastically reducing power consumption, these systems support sustainable technology development. This is particularly critical as industries strive to lower their carbon footprints while maintaining technological growth.
Conclusion
Neuromorphic engineering advancements are revolutionizing AI, providing unprecedented opportunities for innovation across various domains. From healthcare and robotics to smart cities and personalized technology, the potential applications are limitless.
As this transformative field continues to evolve, it promises to deliver more efficient, adaptive, and intelligent systems that align with the needs of a rapidly advancing world. Businesses, researchers, and governments must collaborate to harness the full potential of neuromorphic engineering, shaping a future where technology truly mirrors the brilliance of the human brain.
# Neuromorphic_engineering_advancements #Neuromorphic_chips #Event_driven_processing